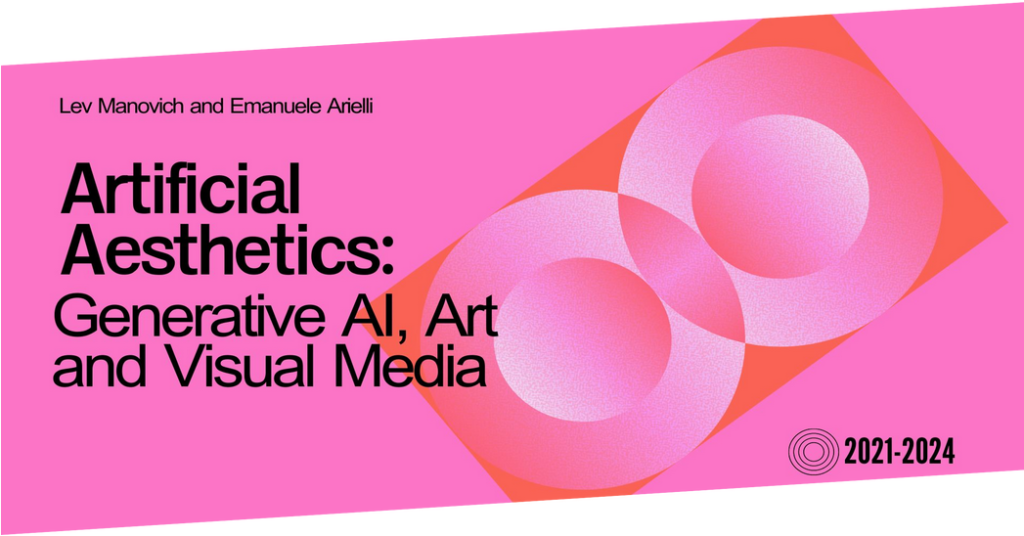
Even an AI can do that (2020) Lev Manovich & Emanuele Arielli
This review is of the first chapter of Artificial Aesthetics: A Critical Guide to AI, Media and Design. The review was completed when the book, by Lev Manovich and Emanuele Arielli, was a work in progress published chapter by chapter online. The area of AI creativity or artificial aesthetics is growing one as machine learning systems have made significant recent advances in the generation of art and design artifacts – with images, designs, and musical compositions in particular. While the text is very much a work in progress with unresolved aspects, it is of importance as Manovich is a significant voice and relatively little has been written on this emerging topic. This intersection of AI and aesthetics is seen as important because aesthetic judgement is seen a quintessentially human domain.
Neural networks (used by Machine Learning/ AI) can be used to abstract aesthetic qualities from large data bases of recorded interactions with aesthetic artifacts, patterns that reveal aesthetic categorisation. If, the chapter asks, ML can extract, describe and categorise all relevant/characteristic features of aesthetic artifacts through advanced pattern recognition, what can we learn about aesthetics from this encounter and from aspects of aesthetics perception that are beyond machine capability?
The authors outline future scenarios where AI systems could, through pattern detection from large data sets, predict what humans will respond to and then generate artifacts that meet these criteria, which could be used as persuasive tools to nudge behaviour (To some extent this has happened since the text was written with the rapid advance of Open AI’s Dall-e and Stability AI’s Stable Diffusion models)
Generation of artifacts, it is argued, will require AI to do cultural analytics and operate evaluatively. This presents issues as applying measurable metrics by which to measure aesthetic response is notoriously unreliable, a brief history of these efforts is outlined. The authors differentiate between experimental aesthetics, small scale psychological tests, and artificial aesthetics, extracting patterns from big data sets, which has advantages over experimental methods as it doesn’t rely on notions of universal aesthetics and selection bias of small focus groups.
While a work in progress without clear conclusions this chapter and the forthcoming book are relevant as new ML tools are set to shape popular ideas of aesthetics and will accelerate moves to assign measurable metrics to aesthetic qualities. This is likely to influence how aesthetics are considered in a variety of pedagogical contexts in technological education and will therefore impact on the outcomes of the project. This suggests that Aesthico needs to engage with these developments.
Conor McGarrigle
TU Dublin
Manovich, Lev, and Emanuele Arielli. “Artificial Aesthetics.” 2024. https://manovich.net/index.php/projects/artificial-aesthetics.